ST-ITO: Controlling Audio Effects for Style Transfer with Inference-Time Optimization
Steinmetz C.J., Singh S., Comunità M., Ibnyahya I., Yuan S., Benetos E., Reiss J.D. - International Society for Music Information Retrieval Conference. ISMIR 2024.
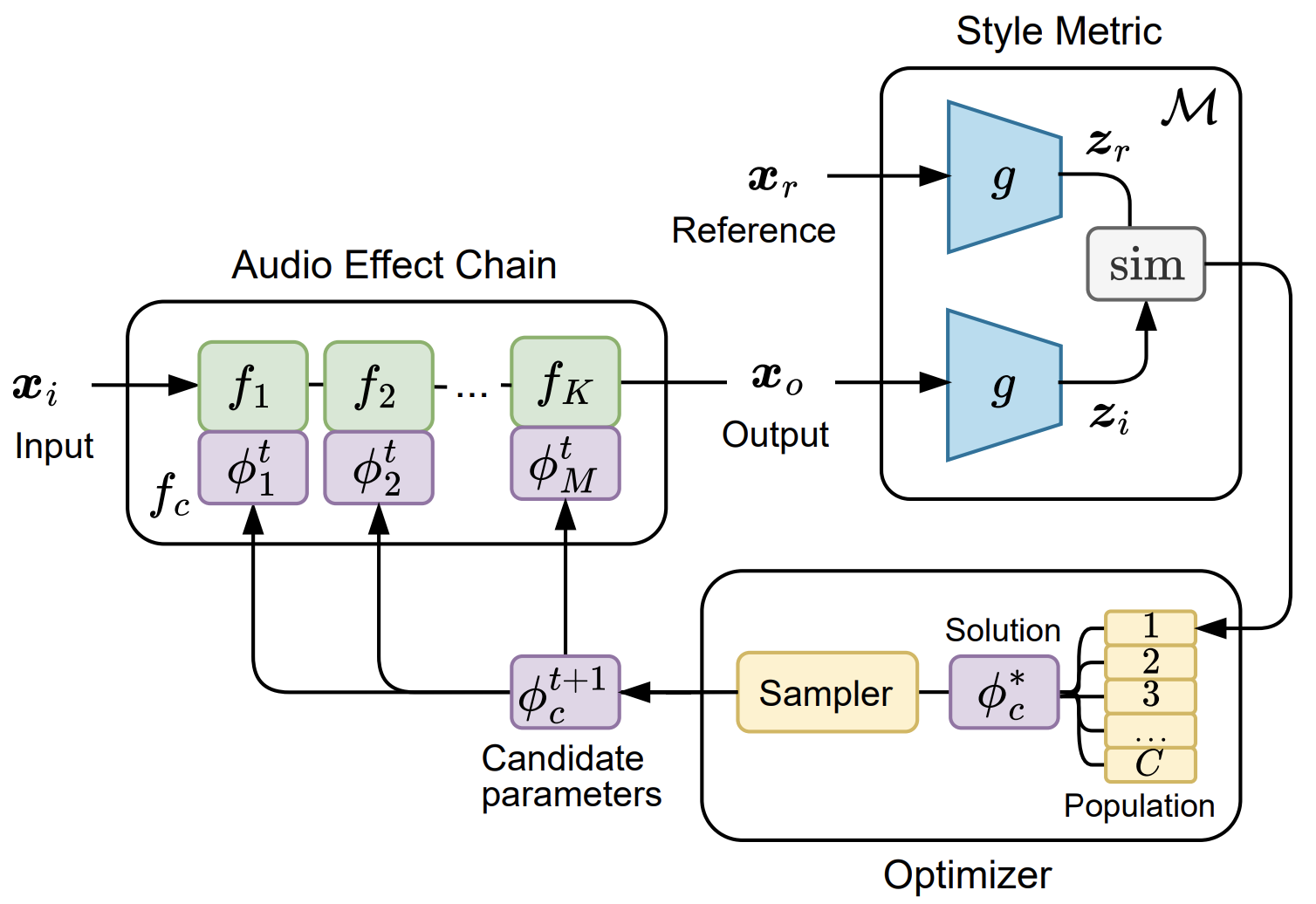
Abstract
Audio production style transfer is the task of processing an input to impart stylistic elements from a reference recording. Existing approaches often train a neural network to estimate control parameters for a set of audio effects. However, these approaches are limited in that they can only control a fixed set of effects, where the effects must be differentiable or otherwise employ specialized training techniques. In this work, we introduce ST-ITO, Style Transfer with Inference-Time Optimization, an approach that instead searches the parameter space of an audio effect chain at inference. This method enables control of arbitrary audio effect chains, including unseen and non-differentiable effects. Our approach employs a learned metric of audio production style, which we train through a simple and scalable self-supervised pretraining strategy, along with a gradient-free optimizer. Due to the limited existing evaluation methods for audio production style transfer, we introduce a multi-part benchmark to evaluate audio production style metrics and style transfer systems. This evaluation demonstrates that our audio representation better captures attributes related to audio production and enables expressive style transfer via control of arbitrary audio effects.
Resources
Citation
Steinmetz C.J., Singh S., Comunità M., Ibnyahya I., Yuan S., Benetos E., Reiss J.D."ST-ITO: Controlling Audio Effects for Style Transfer with Inference-Time Optimization" - International Society for Music Information Retrieval Conference. ISMIR 2024.
- BEST PAPER AWARD -